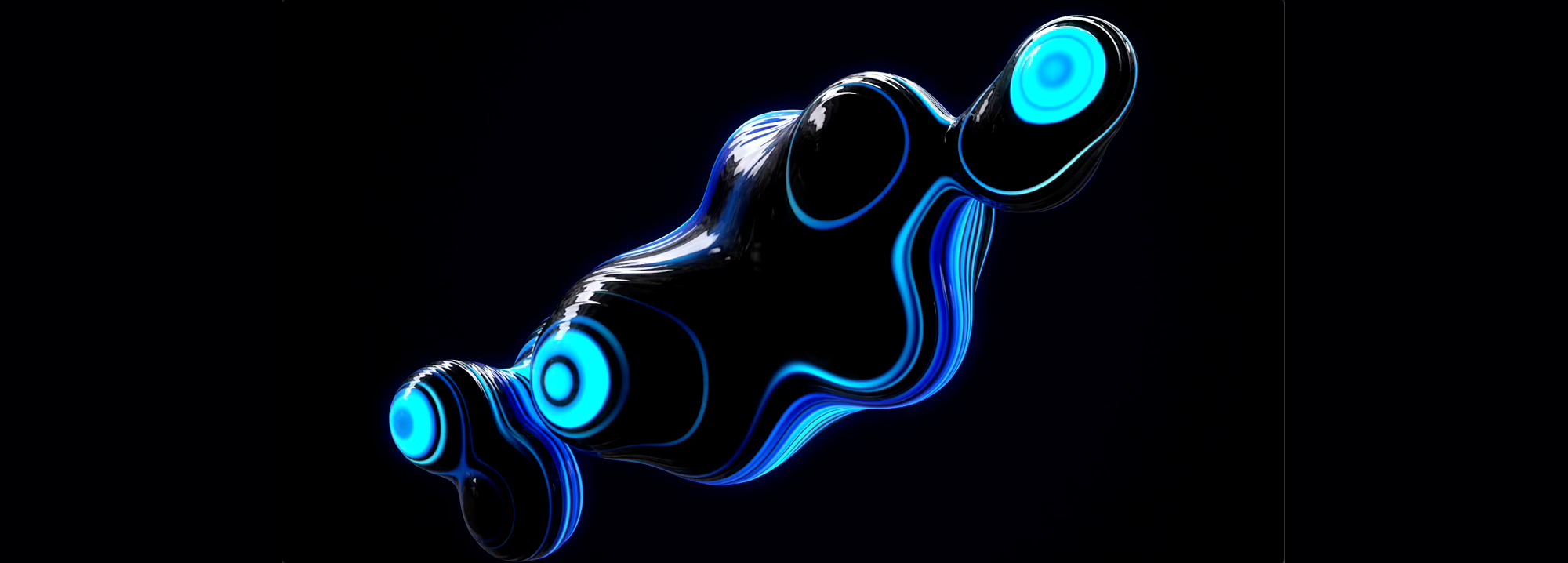
By Tom Khabaza, Principal AI Consultant at Solidatus
For over 30 years, I’ve been helping organizations get more value from their data by using machine learning and data analytics of all kinds. This is about data, yes, but it starts with a business goal, and business knowledge informs every step, so much so that we can define data analysis as the application of knowledge to data. In order to make a useful analysis, the data scientist must use not only knowledge of the data, but also, constantly, knowledge of whatever the data is about, that is knowledge of the business.
Following the recent announcement that Solidatus was named a Representative Vendor in the Gartner® Market Guide for Active Metadata Management, this is the first of four detailed articles on the emerging subject of active metadata. In this blog post, we provide an overview of what it is and the challenges it addresses.
Data and its discontents
Business knowledge is usually found in the minds of businesspeople, so it’s difficult to record, manage and access, and if the data scientist does find a written record of business knowledge, then it’s usually not too suitable for their use, having been created for a different purpose. Data scientists also make use of knowledge that was generated by machine learning, but this is in a machine-usable form, and often not accessible to human examination – machine learning models are usually opaque: they do what they do, but no-one can say what knowledge was used to do it. In any case, the knowledge in a machine learning model is only a tiny part of what is needed to get value from data.
Metadata has the potential and is starting to alleviate these problems by making it easier to record and use both data knowledge and the business knowledge behind it. An application which records the details of the data available to the data scientist, where it can be found, and how it relates to the business, is called a data catalog, and the information it contains is called metadata. This kind of application is invaluable to data scientists, or anyone who has to use data in a complex organization, and several excellent data catalog applications exist.
Metadata – it’s alive!
It is understood less widely that metadata can form more than just a catalog, more than a document in which to look things up. What else can be done with metadata depends on exactly what information is available about the data and about the business processes to which the data relates. If the metadata includes a record of how the data is used, this can be analyzed to improve the use of data – this is the obvious way in which data scientists would use data to improve data science – and this is sometimes called ‘active metadata’. However, metadata can be active in many different ways. If it describes details of how data flows through systems, it can be used to understand the properties of data related to its origin, and to give the organization confidence in downstream systems and data; this is called ‘data lineage’. If the metadata also includes logical information about the data and the business, this can be used automatically to reason and reach conclusions about both the data and the business. All of these properties, plus the dynamic nature of the resultant visualizations, make metadata active, so that it has a direct impact on business decisions, and not only by enabling data analysts.
Active metadata has a curious property, from the point of view of a data scientist. Much of the insight to be gained from metadata comes from treating it as data about individual entities, rather than data about collections, and this makes it easier to visualize, because no aggregation is required. Consider the following diagram, which shows simplified customer and order source system metadata and the metadata for a customer activity report in a data mart:
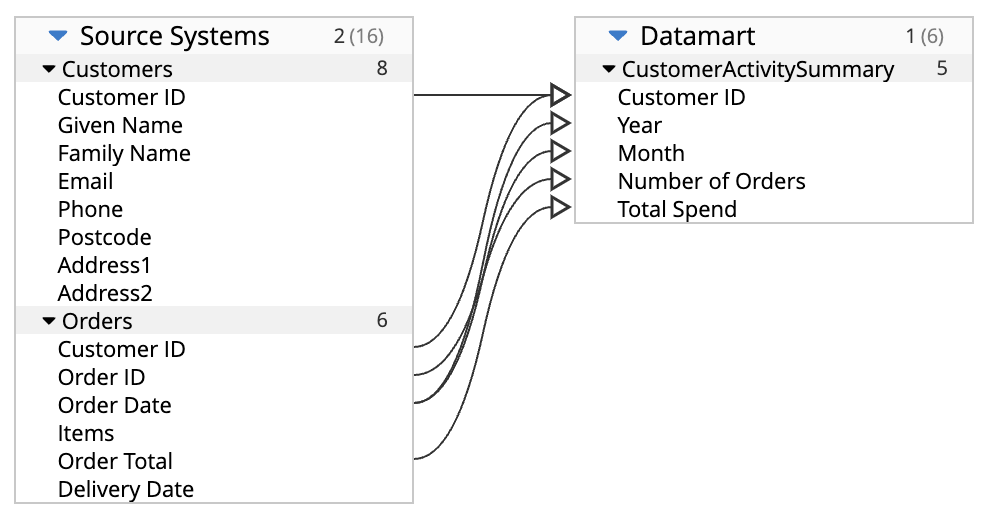
The arrows show data lineage: which source data items contribute to which parts of the report. Data lineage is also metadata, and it’s clear that this kind of metadata is useful if we wish to trace the origins of the report for purposes of trust, or to trace the consequences of proposed changes in the source systems. We might call this ‘technical metadata’ because it describes how pieces of technology fit together, but we can also have ‘business metadata’. Consider the following diagram, which also shows business objects and connections:
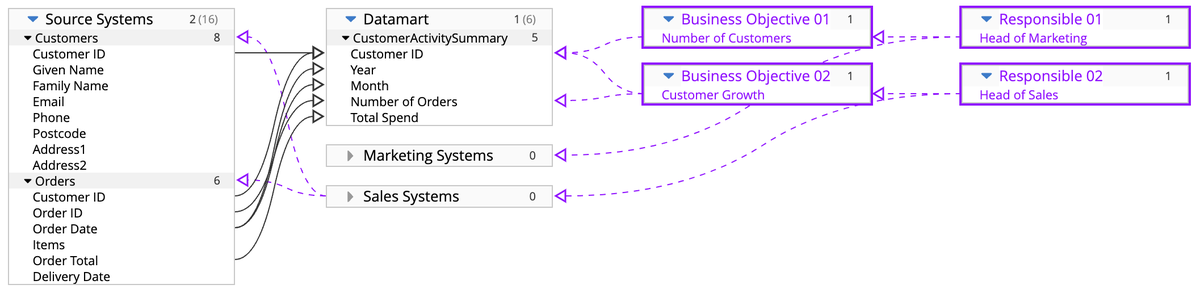
This business metadata allows us to trace relationships across systems, business objectives and responsible managers, and to spot any mismatch between business and technical responsibilities. Note that in all of this metadata, each entity – each database, each data attribute, each objective and each manager – is treated as separate, and insight is gained from chaining together their unique relationships. This is completely different from traditional data analysis, in which insight is gained from aggregations.
Five business challenges solved using metadata
This ability to combine business and technical metadata is hugely powerful, and can be used to solve many different business and data challenges; here are a few examples:
- Governance and regulatory compliance: give an organization unified transparency and automated collaboration across its data, processes and legal obligations, enhancing decision-making and enabling trust in a connected environment.
- Data risk and controls: use an understanding of the flow of data and risk to guide the data lifecycle, retention policies and their privacy implications, in order to reduce both risks and costs.
- Business integration: get a complete view of the business from both technical and management perspectives, and how these are interrelated, enabling joined-up decision-making and clarifying the consequences of proposed changes.
- Data sharing: model all of an organization’s data-related regulations and policies and the data to which they apply, in order to test any data sharing requests and speed up the approval process, enabling more effective insight solutions throughout a complex organization.
- ESG: integrate ESG data into decision-making tools and processes, right-size ESG report automation capabilities to support business growth and evolving regulations, and reduce costs associated with ESG data sourcing, quality assessment, analysis, enrichment and report generation.
All of these are solutions which go beyond localized decision-making; they require a model of the business, its data, the processes that create the data, the controls that monitor it, the policies and standards that guide it, the metrics that measure it and the obligations that regulate it. Furthermore, they require the capability to surface and make visible the results of inference across the business. When metadata provides a rich substrate with integrated inference and dynamic visualization, we call it active metadata.
It’s a whole new world of business improvement and efficiency.
Metadata in action, its business applications, and looking to the future – all yet to come
These are strong claims, and in order to understand and believe them fully, it is necessary to see metadata in action; this will be the topic of the next article in this series. But we need even more than that: we need to understand why metadata can do the things that it does in a business solution, and this will be the focus of the third article, to explain in general terms why metadata offers what it does, and how it can be applied to produce new solutions. The fourth and final article will look to the future: how we can do even more with metadata, and do it more easily, by integrating AI. The future is bright.
Quick Answer: What Is Active Metadata?
In the latest Gartner® report, find out what active metadata is, how to use it, and how to get started.