The value of data: reflections from attending Gartner
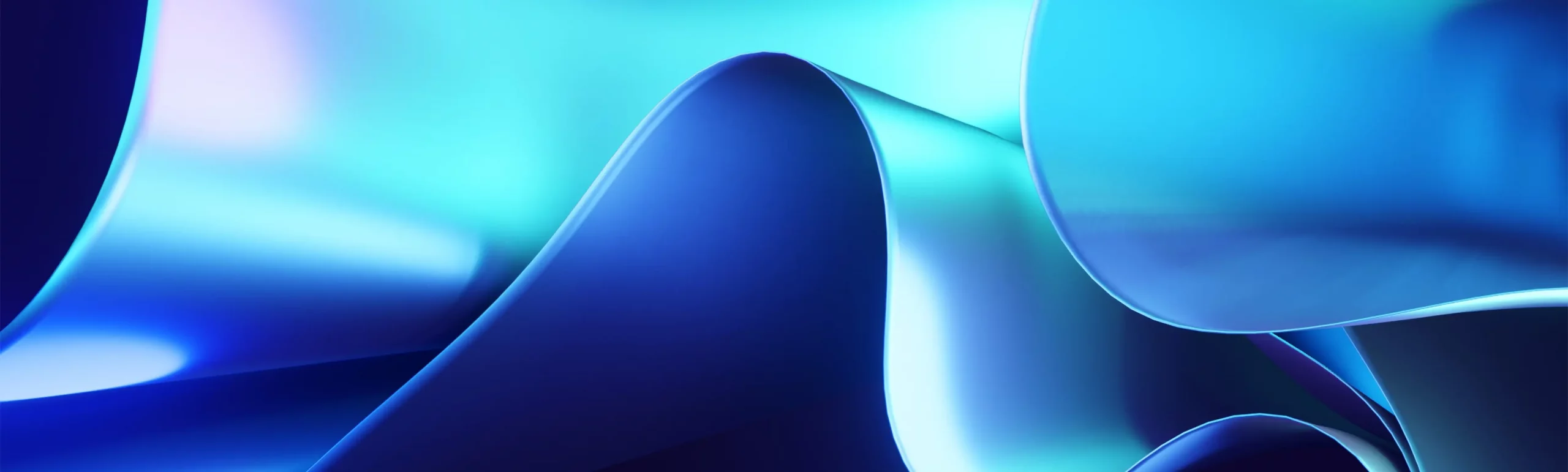
The value of data and other reflections from attending last month’s Gartner® Data & Analytics Summit 2023 in London, U.K.
By Tina Chace, VP Product
That’s me on the far left of the picture above, accompanied by some of my colleagues, including our founder and CEO, Philip Dutton, second from the right.
But my main objective in attending was to listen to people from beyond the Solidatus stand. What was the mood music in this space? And what might I be able to do with it in my new role?
We’ll start with an overview of what I felt were the major themes, these views and observations of mine being an amalgam of the various sessions I attended from a wide variety of speakers and organizations.
Major themes
As a first-time attendee to a data and analytics conference, I observed that:
- Articulating the value of data and governance projects and teams is still challenging. Putting real numbers or quantifying the impact of a governance project can be challenging, and recommendations of talking about financial influencers (such as enabling faster decision-making) should be touted as highly as direct tactical impact, such as headcount reduction. The best examples of getting buy-in from stakeholders outside the data office are real-world use cases which tell the story of value.
- Culture and people are key to a successful data governance organization. There were many examples of success – where an inspirational data leader was able to align technologies, processes and people to achieve their outcomes, and potential pitfalls where there were warnings that purchasing a particular piece of technology isn’t enough if there are no trained data engineers to work in the product and end-users don’t adopt those tools.
- As you’d expect, artificial intelligence was talked about everywhere. It seemed like there was a proliferation of AI/ML/data science (DS) vendors or more traditional vendors that touted how AI/ML was powering their platforms. A significant proportion of sessions had AI as a topic as well. In my view, there are two ways to approach AI through a data and analytics lens: how data governance is a key part of a successful enterprise application of AI and ML, and how AI/ML can assist data and analytics governance. More on this further in the blog.
Let’s expand on these points by rolling the first two into one discussion and finishing with the third on its own.
Data governance programs: the vision
The ideal state for a successful data and governance program is for self-service data products and data governance to be owned by their various domains within a centralized framework – essentially, this dovetails with descriptions I saw of how data fabric architecture and data mesh operating models can be used in combination.
For the more practical data leader, a valuable steppingstone is just breaking the silos between domains to have an accurate map of their own data ecosystem. Our customer, Lewis Reeder from the Bank of New York Mellon, presented with Philip Dutton on the bank’s success in leveraging Solidatus’ metadata layered with business context to clearly visualize their data ecosystem.
One general impression I got from this topic as a whole is of the merits of ensuring that getting to the vision is taken in reasonable and planned increments that each deliver value themselves. The value of understanding your data enables tactical things – such as making the data office operate more efficiently – but also underpins all other functions in the organization. Understanding where your data comes from enables functions such as KYC and AML programs, financial and ESG reporting, algorithmic trading functions, and of course feeding the ML models that help drive operational efficiency and automation in other domains.
The verdict on AI
As discussed in a session entitled The Enterprise Implications of ChatGPT and Generative AI on Monday 22nd May, Gartner has rated techniques such as LLMs (large language models) “not ready for prime time” but something to monitor and research.
I agree wholeheartedly with this judgement based on my past experience of trying to implement them for large and highly regulated institutions. The main limitations are the inability of enterprise customers to safely use and potentially contribute to the open-source datasets that drive models such as OpenAI’s ChatGPT, the effort and responsibility for creating and curating a private dataset while utilizing GPT3/4 technologies to build your own LLMs, and the lack of a big enough use case to make these efforts worth the time and money.
The main use case demonstrated is to enable what I saw called “natural language questioning”. This allows business users to easily ask questions to assist them with self-service data governance like “which systems contain information on customer addresses?” or “what is the impact of adding a new field, tax ID number, to a customer entity?”. I’d describe this as search on steroids.
More traditional machine learning methods, such as classification, matching, data extraction, anomaly detection, and topic modeling, can be used to great effect to support data and analytics governance. Plus, the subject matter expertise will be easier to come by, and implementation is more straightforward.
Classification, for example, can be used to determine whether a system should be controlled by certain procedures based on its properties and relationships to other systems. Matching on both name and semantics can be used to suggest mappings between systems for both technical and business terms to assist in creating and enforcing a centralized data dictionary.
On the flip side, understanding your data ecosystem is key to a successful AI/ML project. From my experience in implementing ML products for regulated enterprise customers, we ran into many roadblocks that a well-mapped understanding of the available data and impacted systems would have solved. Understanding what datasets are available and the quality of the datasets to train your ML model is helpful; there were times when it took days or weeks to approve data to just test one of the ML models we implemented – even on prem. And there were many times when data that could have improved the automation rate of the ML model was excluded because the business stakeholders didn’t know or trust the quality of the data.
Finally, there were many scenarios where changes to upstream systems negatively impacted the performance of ML models and the customer didn’t find out until it had already flowed through.
Putting it into practice
It was great to see so many data leaders talking about their real use cases and success stories. But it was even more interesting to hear about their struggles and what they have learned while implementing their data governance programs.
There was a lot to learn from the panels and sessions but also from talking directly to practising data leaders about their own specific scenarios and pain points. The willingness to share knowledge with peers will surely drive the D&A industry forward as a whole.
I can’t wait to put these insights into practice in my new role.
GARTNER is a registered trademark and service mark of Gartner, Inc. and/or its affiliates in the U.S. and internationally and is used herein with permission. All rights reserved.
Written for Solidatus – a leading data lineage solutions provide.