Douze points for new way to visualize Eurovision data
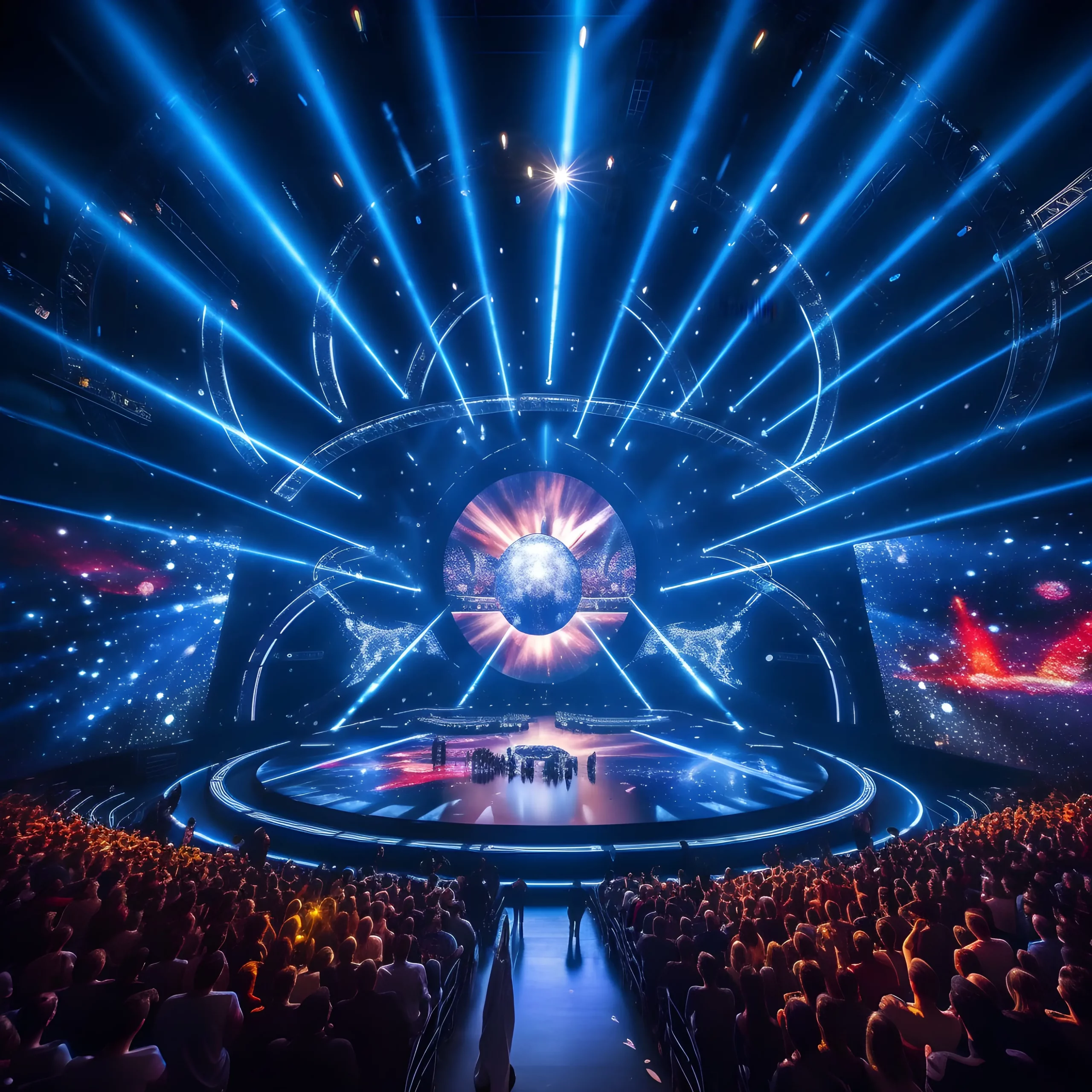
Come closer, come closer and listen.
The beat of my heart keeps on missing.
“Listen to what,” you might reasonably ask, “and, more importantly,” you might go on, “what on earth has your heart got to do with it? Isn’t this a blog about data?”
Well, in a world-first – and, we imagine, much to the joy of 1969-era Lulu – we at Solidatus have linked the Eurovision Song Contest to the realm of data governance and regulatory compliance.
Boom bang-a-bang!
Reality check
“That’s great but so what?”
It’s a fair question. The answer is that this genuinely fascinating work doesn’t just reveal otherwise hard-to-find insights into Eurovision across the ages; the upcoming song contest has provided us with an excuse to develop some models that beautifully illustrate the importance of lineage and how powerful it can be to properly map and visualize data, or rather metadata, whether for business or pleasure.
So back to Eurovision. And in a move that we’re praying won’t equally alienate its two very different target audiences – Eurovision fans and data professionals – we’ve taken a range of rich and granular datasets stretching back to Eurovision’s founding contest in 1956 and fed them into a versatile piece of software that’s more usually used by people working in complex multinational banks and other big businesses.
In this blog post, we’ll:
- Explain how these models work;
- Provide access to them so you can dig around in them yourself;
- Highlight some of our sample findings; and
- Show the parallels between this work and more typical use cases of this software, such as data governance and regulatory compliance.
But first, and to whet your appetite, here are some sample Eurovision findings, upon which we expand later:
- Cyclic voting graphics reveal patterns of pairs of countries each awarding the other top marks – and stats that will be familiar on Greece and Cyprus’s history in this practice.
- In the longest period of consistent voting methodology (1975 to 2015), the highest-scoring winner scored more than three times the points of the lowest-scoring winner, revealing what is arguably the most successful Eurovision song of all time – see who it is below.
- The UK’s belief that it’s getting progressively worse is exaggerated, and we have the analysis to prove it.
But how did we get there? Let’s first take a look at what a Solidatus model is.
A note on Solidatus models
Solidatus models aren’t databases and they don’t store data, or at least not the primary data you’d find in a typical database, with row upon row of similar information on names, addresses, dates of birth etc on thousands of similar records. Rather, they display metadata – data about data – through visualizations that enable users to see how data and systems relate to each other, and how data flows between them, its journey and how it impacts with other data.
In the case of Solidatus, we can meaningfully and with justification describe this metadata as ‘active metadata’, a concept you can read about in our blog post, From data to metadata to active metadata. You can read about other concepts in this field in Key concepts in data governance and managemeny: an A to not-quite-Z guide.
But lest we stray off topic, let’s take a quick look at what a model looks like.
Below is a section of a typical model, not dissimilar to those we’ve used for our Eurovision research. This one, though, was built for a more typical business use case. (We cater for many solutions and sub-solutions.)
A Solidatus model comprises:
- Layers – these are effectively columns. User-defined, they provide a way of grouping together things that belong in the same broad category, whether by time, sequential ‘position’ in a chain of systems or however the user sees fit; in our three Eurovision models, we layer by year, country and placement (see below).
- Objects – these are what you might describe as a primary record. In a normal Solidatus use case, they might represent a database or perhaps a table in a more complex database. Users set their own hierarchy.
- Attributes – these are more granular details of objects.
- Transitions – these are a special type of metadata, shown here as arrowed lines between attributes in different layers, but they’re also recorded in a model’s ‘Relationships’ panel. Typically, they show the flow of data between systems, but they can be used to describe any relationship.
- Properties – these allow you to dig into the weeds of attributes, with information being added or views through the ‘Inspector’ tab on the right-hand side of the screen, or on an object or attribute.
- Relationships – also available through the ‘Inspector’ tab, these show relationship information (transitions) in a tabular form.
When viewing a Solidatus model, bear in mind:
- All of the rich info that can be found in the aforementioned ‘Inspector’ panel, which can be exported into CSV files.
- A range of views are available on the left-hand side, based on what the model builder has built.
- The show trace view and ‘target’ icon will help you isolate flow-related information on specific object and attributes.
- As with our Eurovision models outlined below, skilled model builders might have set up display rules – here, for example we have gold, silver and bronze boxes around entries that were placed first, second and third, and small arrows in country entries that show whether they did better or worse the following year
And finally, a note on how this information is actually brought into Solidatus in the first place:
This is the clever bit. Models can be built manually, and there’s usually manual intervention. But we also have a series of connectors that can automate much of the process.
In the case of this project and alongside some other data repositories, we drew a lot of the info from Wikidata, the central storage area for the structured data of Wikimedia and all the many records on Eurovision it holds. And the connector we built for wider Wikipedia-related metadata ingestion and used for this project is for a query language called SPARQL.
Because here’s the thing: Solidatus doesn’t deal in data that’s unavailable to you if, crucially, you know where to look; rather, it elevates it into a more visually digestible environment, where it can be interrogated in a meaningful context.
But enough of the sales pitch. Let’s dive into the models!
Our three models
By year
This model shows layers arranged by year:
In this view, we’ve scrolled to the right of the screen so that the years 2020, 2021 and 2022 can be seen. But there’s more to the left, going back to 1956, and more below – in each layer, the countries are arranged in descending order of votes garnered.
Here, we’ve clicked on Ukraine in 2022, which shows a transition line from its position the previous year (and the one before that and so on), and changes the focus of the ‘Inspector’ on the right. This panel shows info such as:
- The artist’s name (Kalush);
- The song name (Stefania);
- The language it was sung in (Ukrainian); and
- Its genre(s) (contemporary folk music and, if you can believe it, hip hop).
If a user clicks on ‘Views’ on the left-hand side of the screen, you can also isolate data along the lines shown below, as built by our data modeller.
Cyclic voting, based on who gave whom the top score of douze points, is an interesting one to explore, as illustrated by the transition lines here for the years 2013, 2014 and 2015:
So, when you dig into the model, you can see, for example, that in 2013, Sweden’s judges awarded their two top scores to Denmark and Norway, whereas their entry received only one top score, which they got from Norway’s judges.
By country
This model shows layers arranged by country:
In this view, we’ve scrolled to the left, where we can see the first three countries by alphabetical order – Albania, Andorra and Armenia. By layer, each object is arranged by year, so Albania, for example, first took part in 2004.
At random, we’ve clicked on its record for 2008, the card itself showing that it came 17th that year. The transitions lines pointing into it show which countries (to the right of the model) gave it points. And in the ‘Inspector’, the usual info is available along the lines of the bullets listed in the ‘by year’ model.
‘Zemrën e lamë peng’ was its entry’s catchy title, for example.
By placement
This model shows layers arranged by placement from first to tenth:
Here, we’ve clicked on Sweden’s winning entry in 2015, the transition lines showing whom Sweden gave its votes to and who voted for its winning song, Heroes, sung in English by Måns Zelmerlöw.
And the opportunities for data analysis just go on.
The point of this exercise is to illustrate the richness and granularity of this easily visualizable data, something that of course has more practical applications in the world of big business, rather than to home in on any particular stats. Nonetheless, we feel compelled to highlight a handful of key findings, which you can supplement with your own digging around.
Some sample findings
By choosing the ‘Cyclic Voting (top points)’ view in the ‘by year’ model, we can see that pairs of countries all gave each other 12 points:
- The UK and Switzerland, and Italy and Ireland in 1976, the first year this phenomenon arose;
- Cyprus and Greece in 1986, 1987, 1994, 1997, 1998, 2002, 2003, 2004, 2005, 2010, 2012, 2017 and 2019; and
- Many others we won’t list but you can find yourself, which might raise an eyebrow, given the geopolitical landscape now and in the past.
Cyclic voting in 1976, 1978 and 1979, extracted from a wider model
By exporting the ‘Inspector’ info from the ‘1st’ layer in the ‘by placement’ model, we can see that:
- These are the most successful languages in terms of winning song: English (with 33 wins), Dutch, Hebrew and Italian (all with 3 a piece), German, Norwegian, Serbo-Croat, Spanish, Swedish and Ukrainian (all with 2), and Crimean Tatar, Danish and Portuguese (with 1 each);
- Grand final points for the winning entry have a range of 18 all the way up to Portugal’s barnstorming 758 in 2017, but the huge disparity is in part explained by changing point-awarding scores over the years;
- To conduct a more meaningful analysis, we could look at the years 1975 to 2015, the longest period of consistent methodology, for which the range is 123 (Norway in 1985) to 387 (also Norway, this time in 2009)
- On that last point, with more than three times the score of the lowest-scoring winner in this 40-year period, there’s an argument that Alexander Rybak’s Fairytale (also sung in English) is the ‘best’ song in Eurovision history, although, despite moderate chart success for his song, Rybak is hardly a household name beyond Norway’s shores; and
- The winning song with the shortest title is Netta’s Toy, Israel’s entry for 2018, and the longest title is Poupée de cire, poupée de son, sung by Luxembourg’s France Gall in 1965, who demonstrated that French is sometimes better than English, which would have rendered the song Wax doll, sound doll.
The United Kingdom’s sense that it has done progressively worse in recent years (last year’s second place aside) is exaggerated, given the increasing number of participating countries. The two graphics below show:
- A random portion of the ‘by year’ model, in this case for the period 1964 to 1968, which shows how easy it is to trace the progress – or lineage – of the UK’s performance; and
- A graph derived from this model, which shows the UK’s absolute position alongside a line indicating the percentage of countries that finished above it. While the trend has been downwards, the orange line has been more constant because the number of countries competing has gone up.
Lineage on the UK’s position from 1964 to 1968 inclusive, extracted from a wider model
The UK’s position (blue) and percentage of countries that finished above it (orange) vs year from 1957 to 2022 (with the years it didn’t compete removed)
Lineage, metadata and the world of data compliance
Now, if you’re a data professional with little interest in Eurovision, we’re grateful, frankly, that you’ve stuck with us. Maybe we’ve converted you along the way.
But let’s bring this back to the real world, or at least your world.
The beauty of a visualization tool like Solidatus is that there are virtually no limits to the applications its graph technology can be put to, all of it exploiting and promoting active metadata.
We have, though, found that Solidatus particularly lends itself to these solutions: governance and regulatory compliance; data risk and controls; data sharing; business integration; and environmental, social and governance (ESG).
We’re going to end with a quick review of governance and regulatory compliance.
Using Solidatus, you create living blueprints that map how your data flows – a.k.a. lineage – as it moves through your systems – both now and at other points in time. You can connect your data to the processes that create it, to the policies that guide it, and to the obligations that regulate it. With this framework in place, you can maintain transparency across your business, meet ever-evolving regulatory requirements, and accelerate change programs.
That’s the boilerplate. But what does it mean in practice?
Well, let’s finish with a few excerpts from our recently published case study, Solidatus models HSBC’s global lending book (PDF), this use case – alongside many others, including business integrations, and data risks and controls – being a key component of their several objectives.
In under six months, a team of two was able to document and model the global bank’s entire credit and lending book, demonstrating traceability from source to consumption. They now have a highly scalable and automated solution that is being applied to several applications from ESG to liquidity calculations and other regulatory uses.
Do read the case study (PDF) to see how they reduced a project’s cost from $5,000,000 to under $500,000, a saving of more than 90%.
And don’t let inefficient data management practices be your Waterloo.